Once you’ve gotten started learning R, you can expand your skills by exploring many of the specialized capabilities of R. Here are 6 of the most common areas that people who already have some experience in R find particularly rewarding to learn.
Grab some cheat sheets. No one can possibly remember all the functions and arguments for every R package, which is why cheat sheets were invented. RStudio publishes a free collection of cheat sheets for the most popular R features and packages to help jog your memory. If you decide you’d like to collect them all, you may clone the cheat sheet github repository.
Learn to get help. Everyone gets stuck. Learning where and how to ask for R help is a powerful skill to hone. The Tidyverse site offers some expert advice for how to help others help you. One package you’ll grow to love is the reprex package for creating reproducible R code examples. Read through the reprex articles, which feature loads of animated gifs to illustrate the steps like Magic reprex and Using datapasta with reprex. Where to ask for help? The RStudio Community is a warm and welcoming online discussion forum to ask (and answer!) any questions about using R.
Improve your visualizations. You may already know how to create a basic plot using ggplot2, but can you build one that makes your audience go “Wow?” You can start by expanding your knowledge of the Grammar of Graphics and ggplot2 by reading Hadley Wickham’s (2016) book, ggplot2: Elegant Graphics for Data Analysis. Paper and Kindle versions are available on Amazon for the second edition of the book. The third edition is in-progress and can be viewed for free online, with the source files on GitHub. If you’d like Hadley to personally explain his philosophy of using ggplot2 in his data science work, check out Hadley’s talk from OpenVisConf 2017, The Role of Visualiation in Exploratory Data Analysis. Bookmark the updated R Graphics Cookbook by Winston Chang (2018) too; it is filled with recipes that tackle specific ggplot2 problems.
Develop interactive applications with htmlwidgets and Shiny. One concrete way to communicate your analyses better is to make your visualizations interactive. You can learn how to add browser-based interactivity to your graphics with just a few lines of code at www.htmlwidgets.org. If your interactive needs demand help from R code that needs to run on a server, learn how to write Shiny applications at shiny.rstudio.com, or follow along as Wickham (2020) writes the new Mastering Shiny book. Both approaches can be integrated with R Markdown to create polished interactive dashboards using the flexdashboard package.
Simplify your model explorations with tidymodels. Much of data science involves modeling, but each modeling package seems to invent its own interface and arguments. Enter tidymodels, a meta-package for modeling and analysis that shares the underlying design philosophy, grammar, and data structures of the tidyverse. If you previously have used caret for a uniform modeling interface, the tidymodels package parsnip is its more up-to-date child. While this project is still under development, it promises to dramatically simplify model exploration. RStudio’s Edgar Ruiz wrote up A Gentle Introduction to tidymodels to get you started.
Explore other specialized packages. R attracts data scientists because of its more than 13,000 packages that address nearly every use case. If you’re interested in genomics, you’ll want to spend some time learning the bioconductor collection of packages. If you’re working with Big Data on Spark clusters, check out sparklyr. If you want to dive into finance, you’ll probably want to start with quantmod. To find out what packages you should explore, we recommend some of the topic-based package catalogs such as Awesome R or the CRAN task views.
Tools to help to create tidy data, where each column is a variable, each row is an observation, and each cell contains a single value. Tidyr contains tools for changing the shape (pivoting) and hierarchy (nesting and unnesting) of a dataset, turning deeply nested lists into rectangular data frames (rectangling), and extracting values out of string columns. It also includes tools for working. R Syntax Comparison:: CHEAT SHEET Even within one syntax, there are o'en variations that are equally valid. As a case study, let’s look at the ggplot2 syntax. Ggplot2 is the plotting package that lives within the tidyverse. If you read down this column, all the code here produces the same graphic. Quickplot ggplot. Modelr is stable: it has achieved its goal of making it easier to teach modelling within the tidyverse. For more general modelling tasks, check out the family of “tidymodel” packages like recipes, rsample, parsnip, and tidyposterior.
Bryan, Jennifer, Jim Hester, David Robinson, and Hadley Wickham. 2019. Reprex: Prepare Reproducible Example Code via the Clipboard. https://CRAN.R-project.org/package=reprex.
Chang, Winston. 2018. R Graphics Cookbook: Practical Recipes for Visualizing Data. O’Reilly Media. https://r-graphics.org/.
Chang, Winston, Joe Cheng, JJ Allaire, Yihui Xie, and Jonathan McPherson. 2019. Shiny: Web Application Framework for R. https://CRAN.R-project.org/package=shiny.
Iannone, Richard, JJ Allaire, and Barbara Borges. 2018. Flexdashboard: R Markdown Format for Flexible Dashboards. https://CRAN.R-project.org/package=flexdashboard.
Kuhn, Max, and Davis Vaughan. 2018. Parsnip: A Common Api to Modeling and Analysis Functions. https://CRAN.R-project.org/package=parsnip.
Max, Kuhn, and Hadley Wickham. 2018. Tidymodels: Easily Install and Load the ’Tidymodels’ Packages. https://CRAN.R-project.org/package=tidymodels.
Ryan, Jeffrey A., and Joshua M. Ulrich. 2018. Quantmod: Quantitative Financial Modelling Framework. https://CRAN.R-project.org/package=quantmod.
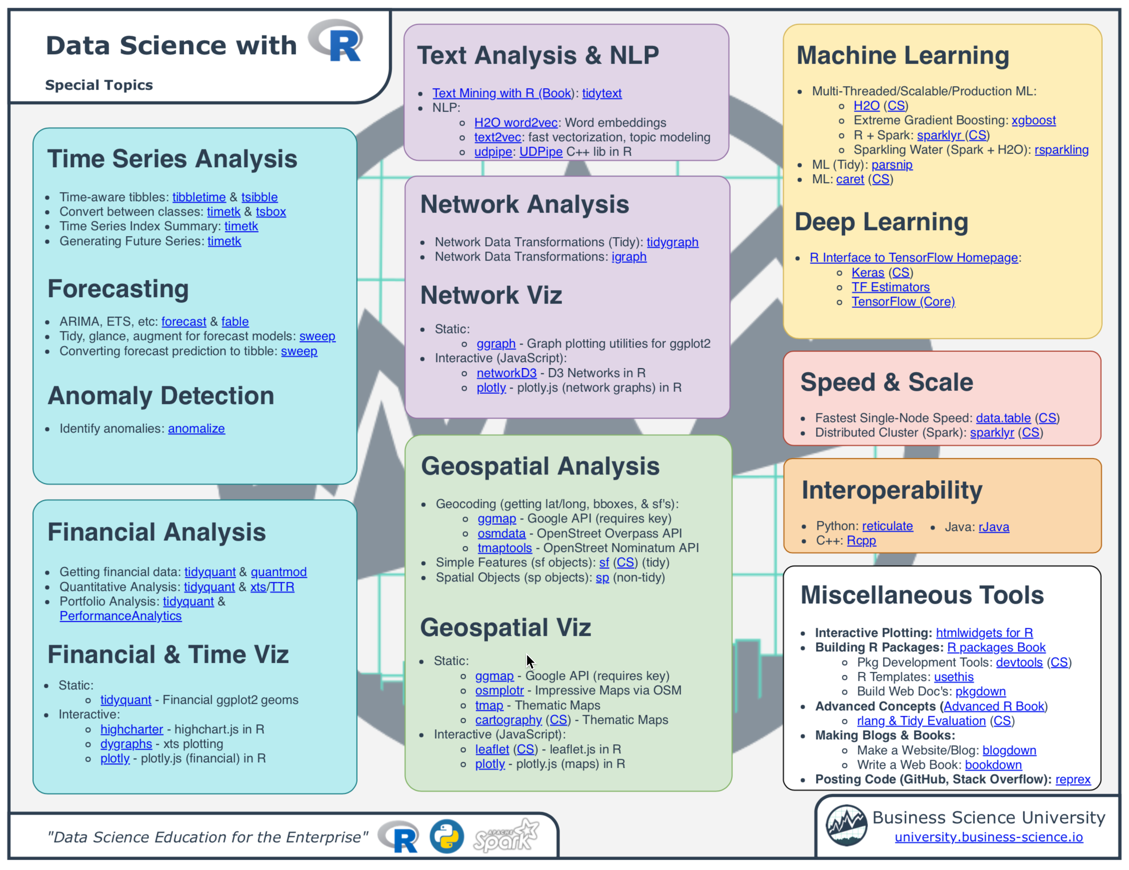
Wickham, Hadley. 2016. Ggplot2: Elegant Graphics for Data Analysis. Springer. https://ggplot2-book.org/.
———. 2020. Mastering Shiny. O’Reilly Media. https://mastering-shiny.org/.
Wickham, Hadley, Winston Chang, Lionel Henry, Thomas Lin Pedersen, Kohske Takahashi, Claus Wilke, Kara Woo, and Hiroaki Yutani. 2019. Ggplot2: Create Elegant Data Visualisations Using the Grammar of Graphics.
Approximate time: 75 minutes
The Tidyverse suite of integrated packages are designed to work together to make common data science operations more user friendly. The packages have functions for data wrangling, tidying, reading/writing, parsing, and visualizing, among others. There is a freely available book, R for Data Science, with detailed descriptions and practical examples of the tools available and how they work together. We will explore the basic syntax for working with these packages, as well as, specific functions for data wrangling with the ‘dplyr’ package, data tidying with the ‘tidyr’ package, and data visualization with the ‘ggplot2’ package.
All of these packages use the same style of code, which is snake_case
formatting for all function names and arguments. The tidy style guide is available for perusal.
Adding files to your working directory
We have three files that we need to bring in for this lesson:
- A normalized counts file (gene expression counts normalized for library size)
- A metadata file corresponding to the samples in our normalized counts dataset
- The differential expression results output from our DE analysis using DESeq2
Download the files to the data
folder by right-clicking the links below:
- Normalized counts file: right-click here
- Differential expression results: right-click here
Choose to Save Link As
or Download Linked File As
and navigate to your Visualizations-in-R/data
folder. You should now see the files appear in the data
folder in the RStudio file directory.
Reading in the data files
Let’s read in all of the files we have downloaded:
Tidyverse basics
As it is difficult to change how fundamental base R structures/functions work, the Tidyverse suite of packages create and use data structures, functions and operators to make working with data more intuitive. The two most basic changes are in the use of pipes and tibbles.
Pipes
Stringing together commands in R can be quite daunting. Also, trying to understand code that has many nested functions can be confusing.
To make R code more human readable, the Tidyverse tools use the pipe, %>%
, which was acquired from the ‘magrittr’ package and comes installed automatically with Tidyverse. The pipe allows the output of a previous command to be used as input to another command instead of using nested functions.
NOTE: Shortcut to write the pipe is shift + command + M
An example of using the pipe to run multiple commands:
The pipe represents a much easier way of writing and deciphering R code, and we will be taking advantage of it for all future activities.
Exercises
Extract the
replicate
column from themetadata
data frame (use the$
notation) and save the values to a vector namedrep_number
.Use the pipe (
%>%
) to perform two steps in a single line:- Turn
rep_number
into a factor. - Use the
head()
function to return the first six values of therep_number
factor.
- Turn
Tibbles
A core component of the tidyverse is the tibble. Tibbles are a modern rework of the standard data.frame, with some internal improvements to make code more reliable. They are data frames, but do not follow all of the same rules. For example, tibbles can have column names that are not normally allowed, such as numbers/symbols.
Important: tidyverse is very opininated about row names. These packages insist that all column data (e.g. data.frame
) be treated equally, and that special designation of a column as rownames
should be deprecated. Tibble provides simple utility functions to handle rownames: rownames_to_column()
and column_to_rownames()
. More help for dealing with row names in tibbles can be found:
Tibbles can be created directly using the tibble()
function or data frames can be converted into tibbles using as_tibble(name_of_df)
.
NOTE: The function as_tibble()
will ignore row names, so if a column representing the row names is needed, then the function rownames_to_column(name_of_df)
should be run prior to turning the data.frame into a tibble. Also, as_tibble()
will not coerce character vectors to factors by default.
Exercises
Create a tibble called
df_tibble
using thetibble()
function to combine the vectorsspecies
andglengths
.Change the
metadata
data frame to a tibble calledmeta_tibble
. Use therownames_to_column()
function to preserve the rownames combined with using%>%
and theas_tibble()
function.
Differences between tibbles and data.frames
The main differences between tibbles and data.frames relate to printing and subsetting.
Printing
A nice feature of a tibble is that when printing a variable to screen, it will show only the first 10 rows and the columns that fit to the screen by default. This is nice since you don’t have to specify head to take a quick look at your dataset. If it is desirable to view more of the dataset, the print()
function can change the number of rows or columns displayed.
Subsetting
When subsetting base R data.frames the default behavior is to simplify the output to the simplest data structure. Therefore, if subsetting a single column from a data.frame, R will output a vector (unless drop=FALSE
is specified). In contrast, subsetting a single column of a tibble will by default return another tibble, not a vector.
Due to this behavior, some older functions do not work with tibbles, so if you need to convert a tibble to a data.frame, the function as.data.frame(name_of_tibble)
will easily convert it.
Also note that if you use piping to subset a data frame, then the notation is slightly different, requiring a placeholder .
prior to the [ ]
or $
.
Tidyverse tools
While all of the tools in the Tidyverse suite are deserving of being explored in more depth, we are going to investigate only the tools we will be using most for data wrangling and tidying.
Dplyr
The most useful tool in the tidyverse is dplyr. It’s a swiss-army knife for data wrangling. dplyr has many handy functions that we recommend incorporating into your analysis:
select()
extracts columns and returns a tibble.arrange()
changes the ordering of the rows.filter()
picks cases based on their values.mutate()
adds new variables that are functions of existing variables.rename()
easily changes the name of a column(s)summarise()
reduces multiple values down to a single summary.pull()
extracts a single column as a vector._join()
group of functions that merge two data frames together, includes (inner_join()
,left_join()
,right_join()
, andfull_join()
).
Note:dplyr underwent a massive revision this year, switching versions from 0.5 to 0.7. If you consult other dplyr tutorials online, note that many materials developed prior to 2017 are no longer correct. In particular, this applies to writing functions with dplyr (see Notes section below).
select()
To extract columns from a tibble we can use the select()
.
Conversely, you can remove columns you don’t want with negative selection.
arrange()
Note that the rows are sorted by the gene symbol. Let’s fix that and sort them by adjusted P value instead with arrange()
.
filter()
Let’s keep only genes that are expressed (baseMean
above 0) with an adjusted P value below 0.01. You can perform multiple filter()
operations together in a single command.
mutate()
mutate()
enables you to create a new column from an existing column. Let’s generate log10 calculations of our baseMeans for each gene.
rename()
You can quickly rename an existing column with rename()
. The syntax is new_name
= old_name
.
summarise()
You can perform column summarization operations with summarise()
.
Advanced:summarise()
is particularly powerful in combination with the group_by()
function, which allows you to group related rows together.
Note: summarize()
also works if you prefer to use American English. This applies across the board to any tidy functions, including in ggplot2 (e.g. color
in place of colour
).
pull()
In the recent dplyr 0.7 update, pull()
was added as a quick way to access column data as a vector. This is very handy in chain operations with the pipe operator.
_join()
Dplyr has a powerful group of join operations, which join together a pair of data frames based on a variable or set of variables present in both data frames that uniquely identify all observations. These variables are called keys.
inner_join
: Only the rows with keys present in both datasets will be joined together.left_join
: Keeps all the rows from the first dataset, regardless of whether in second dataset, and joins the rows of the second that have keys in the first.right_join
: Keeps all the rows from the second dataset, regardless of whether in first dataset, and joins the rows of the first that have keys in the second.full_join
: Keeps all rows in both datasets. Rows without matching keys will have NA values for those variables from the other dataset.
To practice with the join functions, we can use a couple of built-in R datasets.
Tidyr
The purpose of Tidyr is to have well-organized or tidy data, which Tidyverse defines as having:
- Each variable in a column
- Each observation in a row
- Each value as a cell
There are two main functions in Tidyr, gather()
and spread()
. These functions allow for conversion between long data format and wide data format. The downstream use of the data will determine which format is required.
gather()
The gather()
function changes a wide data format into a long data format. This function is particularly helpful when using ‘ggplot2’ to get all of the values to plot into a single column.
To use this function, you need to give the columns in the data frame you would like to gather together as a single column. Then, provide a name to give the column where all of the column names will be present using the key
argument, and the name to give the column where all of the values will be present using the value
argument.
spread()
The spread()
function is the reverse of the gather()
function. The categories of the key
column will become separate columns, and the values in the value
column split across the associated key
columns.
R Data Cleaning Cheat Sheet
Programming notes
Tidyr Cheat Sheet
Underneath the hood, tidyverse packages build upon the base R language using rlang, which is a complete rework of how functions handle variable names and evaluate arguments. This is achieved through the tidyeval
framework, which interprates command operations using tidy evaluation
. This is outside of the scope of the course, but explained in detail in the Programming with dplyr vignette, in case you’d like to understand how these new tools behave differently from base R.
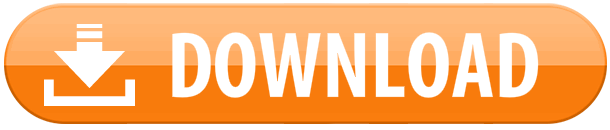